Dear Stephane,
First of all, I really appreciate your effort in this wonderful package.
I want to use the ordered logit model for the choice probability part instead of MNL because my choice part is a Likert scale from 1 to 5.
However, the example code of ICLV is for MNL, so I need to revise some.
Can you help me with how to revise it? I wanted to use the ordered logit part instead of MNL in the choice part,
but I am afraid of integrated likelihood function difference or the alternative since I have one column for respondences' preference.
How can I revise the choice part?
Thank you in advance.
With best regards,
Hyewon
Important: Read this before posting to this forum
- This forum is for questions related to the use of Apollo. We will answer some general choice modelling questions too, where appropriate, and time permitting. We cannot answer questions about how to estimate choice models with other software packages.
- There is a very detailed manual for Apollo available at http://www.ApolloChoiceModelling.com/manual.html. This contains detailed descriptions of the various Apollo functions, and numerous examples are available at http://www.ApolloChoiceModelling.com/examples.html. In addition, help files are available for all functions, using e.g. ?apollo_mnl
- Before asking a question on the forum, users are kindly requested to follow these steps:
- Check that the same issue has not already been addressed in the forum - there is a search tool.
- Ensure that the correct syntax has been used. For any function, detailed instructions are available directly in Apollo, e.g. by using ?apollo_mnl for apollo_mnl
- Check the frequently asked questions section on the Apollo website, which discusses some common issues/failures. Please see http://www.apollochoicemodelling.com/faq.html
- Make sure that R is using the latest official release of Apollo.
- Users can check which version they are running by entering packageVersion("apollo").
- Then check what is the latest full release (not development version) at http://www.ApolloChoiceModelling.com/code.html.
- To update to the latest official version, just enter install.packages("apollo"). To update to a development version, download the appropriate binary file from http://www.ApolloChoiceModelling.com/code.html, and install the package from file
- If the above steps do not resolve the issue, then users should follow these steps when posting a question:
- provide full details on the issue, including the entire code and output, including any error messages
- posts will not immediately appear on the forum, but will be checked by a moderator first. This may take a day or two at busy times. There is no need to submit the post multiple times.
How can I integrate ordered logit instead of MNL in ICLV?
-
- Site Admin
- Posts: 1042
- Joined: 24 Apr 2020, 16:29
Re: How can I integrate ordered logit instead of MNL in ICLV?
Hi Hyewon
this is quite easy to do. You just need to replace the mnl_settings part by the appropriate settings for ol_settings, and then use apollo_ol instead of apollo_mnl
Maybe you could have a first attempt at setting this up yourself and then show me where you get to
Thanks
Stephane
this is quite easy to do. You just need to replace the mnl_settings part by the appropriate settings for ol_settings, and then use apollo_ol instead of apollo_mnl
Maybe you could have a first attempt at setting this up yourself and then show me where you get to
Thanks
Stephane
Re: How can I integrate ordered logit instead of MNL in ICLV?
Dear Stephane,
Thank you for your prompt reply.
As I said before, I want to use ordered logit instead of MNL in the choice part in ICLV.
I used ol_setting instead of MNL_setting in the utility part,
it somehow works, but some of the results give n/a though it should have the value.
Therefore, the problem is the code. It will be really appreciated if you can give the comments on the problem.
I will explain the detail of my assumption in detail as well as the code.
First, I will attach the framework of the model that I thought.
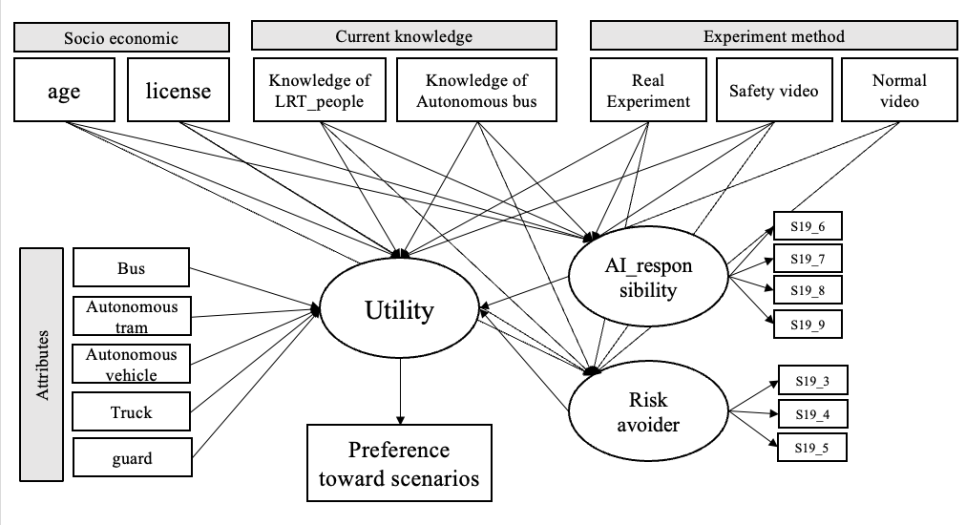
Second, I will briefly explain the name that I used in the code.
There are two latent variables, "ai" and "risk". Those are Likert scales.
For the choice part, it named "att" in the code. Also Likert scale, the tau is "tau_choice"
I also add some explanation in the beta definition part for your understanding
Here, the code which I utilize is as follows;
-----------------------------------------------------------------------------------------------
Some parameters show unavailable values. I will be really appreciated it if you give some comments on where I need to revise.
Thank you so much for the best tool for choice modeling, again.
With best regards,
Hyewon
Thank you for your prompt reply.
As I said before, I want to use ordered logit instead of MNL in the choice part in ICLV.
I used ol_setting instead of MNL_setting in the utility part,
it somehow works, but some of the results give n/a though it should have the value.
Therefore, the problem is the code. It will be really appreciated if you can give the comments on the problem.
I will explain the detail of my assumption in detail as well as the code.
First, I will attach the framework of the model that I thought.
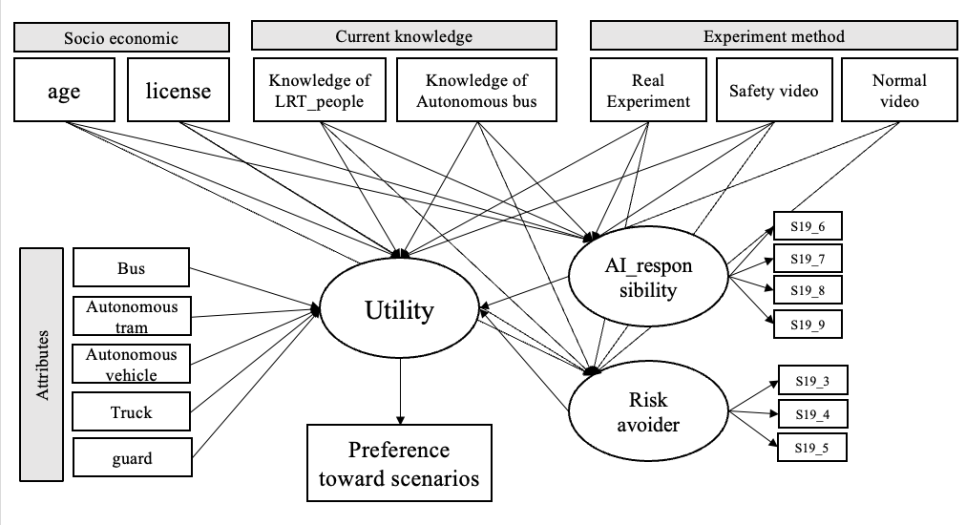
Second, I will briefly explain the name that I used in the code.
There are two latent variables, "ai" and "risk". Those are Likert scales.
For the choice part, it named "att" in the code. Also Likert scale, the tau is "tau_choice"
I also add some explanation in the beta definition part for your understanding
Here, the code which I utilize is as follows;
-----------------------------------------------------------------------------------------------
Code: Select all
# ################################################################# #
#### DEFINE MODEL PARAMETERS ####
# ################################################################# #
### Vector of parameters, including any that are kept fixed in estimation
apollo_beta = c(
# attributes for choice part
gamma_tram = 0,
gamma_auto_tram = 0,
gamma_auto_vehi = 0,
gamma_bus = 0,
gamma_truck_person = 0,
gamma_truck_guard = 0,
# lambda_ai = 1,
#lambda_risk = 1,
# socio-economic variables for choice part
gamma_age = 0,
gamma_license = 0,
gamma_k_tram = 0, #knowledge of tram
gamma_k_social = 0, #knowledge of social experiment
gamma_experi = 0, # experiment method, binary
gamma_video = 0, # experiment method, binary
gamma_none = 0,
# socio-economic of latent variable part
gamma_ai_age = 0,
gamma_ai_license = 0,
gamma_ai_k_tram = 0,
gamma_ai_k_social = 0,
gamma_ai_experi = 0,
gamma_ai_video = 0,
gamma_ai_none = 0,
gamma_risk_age = 0,
gamma_risk_license = 0,
gamma_risk_experi = 0,
gamma_risk_video = 0,
gamma_risk_none = 0,
# tau for choice part (choice part is using ordered logit)
tau_choice_1 = -2,
tau_choice_2 = -1,
tau_choice_3 = 1,
tau_choice_4 = 2,
# tau for latent variables
zeta_ai_1 = 1,
zeta_ai_2 = 0,
zeta_ai_3 = 0,
zeta_ai_4 = 0,
tau_ai1_1 =-2,
tau_ai1_2 =-1,
tau_ai1_3 = 1,
tau_ai1_4 = 2,
tau_ai2_1 =-2,
tau_ai2_2 =-1,
tau_ai2_3 = 1,
tau_ai2_4 = 2,
tau_ai3_1 =-2,
tau_ai3_2 =-1,
tau_ai3_3 = 1,
tau_ai3_4 = 2,
tau_ai4_1 =-2,
tau_ai4_2 =-1,
tau_ai4_3 = 1,
tau_ai4_4 = 2,
zeta_risk_1 = 1,
zeta_risk_2 = 0,
zeta_risk_3 = 0,
tau_risk1_1 =-2,
tau_risk1_2 =-1,
tau_risk1_3 = 1,
tau_risk1_4 = 2,
tau_risk2_1 =-2,
tau_risk2_2 =-1,
tau_risk2_3 = 1,
tau_risk2_4 = 2,
tau_risk3_1 =-2,
tau_risk3_2 =-1,
tau_risk3_3 = 1,
tau_risk3_4 = 2
)
### Vector with names (in quotes) of parameters to be kept fixed at their starting value in apollo_beta, use apollo_beta_fixed = c() if none
apollo_fixed = c()
# ################################################################# #
#### DEFINE RANDOM COMPONENTS ####
# ################################################################# #
### Set parameters for generating draws
apollo_draws = list(
interDrawsType="halton",
interNDraws=100,
interUnifDraws=c(),
interNormDraws=c("eta_ai", "eta_risk", "eta_att"),
intraDrawsType='',
intraNDraws=0,
intraUnifDraws=c(),
intraNormDraws=c()
)
### Create random parameters
apollo_randCoeff=function(apollo_beta, apollo_inputs){
randcoeff = list()
randcoeff[["LV_ai"]] = gamma_ai_age*age + gamma_ai_license*license + gamma_ai_k_tram*k_tram +
gamma_ai_k_social*k_social + gamma_ai_video*video1 + gamma_ai_none*video2 + gamma_ai_experi*exp_group+ eta_ai
randcoeff[["LV_risk"]] = gamma_risk_age*age + gamma_risk_license*license + gamma_risk_video*video1 +
gamma_risk_none*video2 + gamma_risk_experi*exp_group + eta_risk
randcoeff[["LV_att"]] = gamma_tram*tram + gamma_auto_tram*auto_tram + gamma_auto_vehi*auto_vehi + gamma_bus*bus +
gamma_truck_person*truck_person + gamma_truck_guard*truck_guard + gamma_age* age + gamma_license*license +
gamma_k_tram*k_tram + gamma_k_social*k_social + gamma_experi*exp_group + gamma_none*video2 + gamma_video*video1 +eta_att
return(randcoeff)
}
# ################################################################# #
#### GROUP AND VALIDATE INPUTS ####
# ################################################################# #
apollo_inputs = apollo_validateInputs()
# ################################################################# #
#### DEFINE MODEL AND LIKELIHOOD FUNCTION ####
# ################################################################# #
apollo_probabilities=function(apollo_beta, apollo_inputs, functionality="estimate"){
### Attach inputs and detach after function exit
apollo_attach(apollo_beta, apollo_inputs)
on.exit(apollo_detach(apollo_beta, apollo_inputs))
### Create list of probabilities P
P = list()
### Likelihood of indicators
ol_settings1 = list(outcomeOrdered = s19_6,
V = zeta_ai_1*LV_ai,
tau = c(tau_ai1_1, tau_ai1_2, tau_ai1_3, tau_ai1_4),
rows = (task==1))
ol_settings2 = list(outcomeOrdered = s19_7,
V = zeta_ai_2*LV_ai,
tau = c(tau_ai2_1, tau_ai2_2, tau_ai2_3, tau_ai2_4),
rows = (task==1))
ol_settings3 = list(outcomeOrdered = s19_8,
V = zeta_ai_3*LV_ai,
tau = c(tau_ai3_1, tau_ai3_2, tau_ai3_3, tau_ai3_4),
rows = (task==1))
ol_settings4 = list(outcomeOrdered = s19_9,
V = zeta_ai_4*LV_ai,
tau = c(tau_ai4_1, tau_ai4_2, tau_ai4_3, tau_ai4_4),
rows = (task==1))
ol_settings5 = list(outcomeOrdered = s20_3,
V = zeta_risk_1*LV_risk,
tau = c(tau_risk1_1, tau_risk1_2, tau_risk1_3, tau_risk1_4),
rows = (task==1))
ol_settings6 = list(outcomeOrdered = s20_4,
V = zeta_risk_2*LV_risk,
tau = c(tau_risk2_1, tau_risk2_2, tau_risk2_3, tau_risk2_4),
rows = (task==1))
ol_settings7 = list(outcomeOrdered = s20_5,
V = zeta_risk_3*LV_risk,
tau = c(tau_risk3_1, tau_risk3_2, tau_risk3_3, tau_risk3_4),
rows = (task==1))
P[["ai_1"]] = apollo_ol(ol_settings1, functionality)
P[["ai_2"]] = apollo_ol(ol_settings2, functionality)
P[["ai_3"]] = apollo_ol(ol_settings3, functionality)
P[["ai_4"]] = apollo_ol(ol_settings4, functionality)
P[["risk_1"]] = apollo_ol(ol_settings5, functionality)
P[["risk_2"]] = apollo_ol(ol_settings6, functionality)
P[["risk_3"]] = apollo_ol(ol_settings7, functionality)
### Likelihood of choices
### List of utilities: these must use the same names as in ol_settings, order is irrelevant
ol_settings = list(outcomeOrdered = choice,
V = LV_att*LV_risk*LV_ai,
tau = c(tau_choice_1, tau_choice_2, tau_choice_3, tau_choice_4),
componentName = "choice_part")
### Compute ordered logit probabilites for choice part
P[["choice"]] = apollo_ol(ol_settings, functionality)
### Likelihood of the whole model
P = apollo_combineModels(P, apollo_inputs, functionality)
### Take product across observation for same individual
P = apollo_panelProd(P, apollo_inputs, functionality)
### Prepare and return outputs of function
#P = apollo_prepareProb(P, apollo_inputs, functionality)
### Compute probabilities for MNL model component
# P[["model"]] = apollo_mnl(mnl_settings, functionality)
### Average across inter-individual draws
P = apollo_avgInterDraws(P, apollo_inputs, functionality)
### Prepare and return outputs of function
P = apollo_prepareProb(P, apollo_inputs, functionality)
return(P)
}
# ################################################################# #
#### MODEL ESTIMATION ####
# ################################################################# #
### Optional: calculate LL before model estimation
# apollo_llCalc(apollo_beta, apollo_probabilities, apollo_inputs)
### Estimate model
model = apollo_estimate(apollo_beta, apollo_fixed, apollo_probabilities, apollo_inputs)
# ################################################################# #
#### MODEL OUTPUTS ####
# ################################################################# #
# ----------------------------------------------------------------- #
#---- FORMATTED OUTPUT (TO SCREEN) ----
# ----------------------------------------------------------------- #
apollo_modelOutput(model)
Some parameters show unavailable values. I will be really appreciated it if you give some comments on where I need to revise.
Thank you so much for the best tool for choice modeling, again.
With best regards,
Hyewon
-
- Site Admin
- Posts: 1042
- Joined: 24 Apr 2020, 16:29
Re: How can I integrate ordered logit instead of MNL in ICLV?
Hi
Can you please also share the output so we can see what's going wrong?
Thanks
Stephane
Can you please also share the output so we can see what's going wrong?
Thanks
Stephane
Re: How can I integrate ordered logit instead of MNL in ICLV?
Dear Stephane,
This is Hyewon, again.
I would add the result of the code also the framework of the model, again.
I added the image last time, but it does not show, so I will add it again.
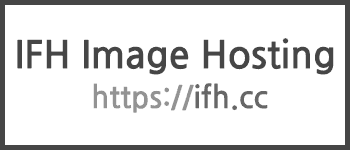
The result of the model is shown as follows
I ran three different datasets, but some show NaN in different values, in t-stat and SE.
I think this might be a problem of the code, and also I am not sure about the integrating latent variables and choice (ordered logit) part,
"Likelihood of choices" part, and generating multiple latent variables,
I will really appreciate your guidance,
Thank you in advance.
With best regards,
Hyewon
This is Hyewon, again.
I would add the result of the code also the framework of the model, again.
I added the image last time, but it does not show, so I will add it again.
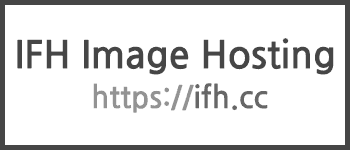
The result of the model is shown as follows
Code: Select all
Model name : ICLV for conference_group3
Model description : ICLV model on bus-tram connected with (non)experiment groups
Model run at : 2021-04-07 10:17:05
Estimation method : bfgs
Model diagnosis : successful convergence
Number of individuals : 988
Number of rows in database : 8892
Number of modelled outcomes : 15808
ai_1: 988
ai_2: 988
ai_3: 988
ai_4: 988
choice_part: 8892
risk_1: 988
risk_2: 988
risk_3: 988
Number of cores used : 3
Number of inter-individual draws : 100 (halton)
LL(start) : -23738.18
LL(0, whole model) : Not applicable
LL(final, whole model) : -17179.12
Rho-square (0) : Not applicable
Adj.Rho-square (0) : Not applicable
AIC : 34486.24
BIC : 34977.01
LL(0,ai_1) : Not applicable
LL(final,ai_1) : -1232.157
LL(0,ai_2) : Not applicable
LL(final,ai_2) : -1202.419
LL(0,ai_3) : Not applicable
LL(final,ai_3) : -1205.084
LL(0,ai_4) : Not applicable
LL(final,ai_4) : -1220.752
LL(0,risk_1) : Not applicable
LL(final,risk_1) : -1416.25
LL(0,risk_2) : Not applicable
LL(final,risk_2) : -1233.975
LL(0,risk_3) : Not applicable
LL(final,risk_3) : -1290.342
LL(0,choice) : Not applicable
LL(final,choice) : -9914.957
Estimated parameters : 64
Time taken (hh:mm:ss) : 00:56:17.55
pre-estimation : 00:00:17.75
estimation : 00:33:10.44
post-estimation : 00:22:49.35
Iterations : 157
Min abs eigenvalue of Hessian : 0.001955
Some eigenvalues of Hessian are positive, indicating potential problems!
Estimates:
Estimate s.e. t.rat.(0) Rob.s.e. Rob.t.rat.(0)
gamma_tram 2.260637 NaN NaN 0.674486 3.3516
gamma_auto_tram 2.284710 NaN NaN 0.640076 3.5694
gamma_auto_vehi 0.464076 0.093246 4.9769 0.238847 1.9430
gamma_bus 0.298343 0.098243 3.0368 0.326497 0.9138
gamma_truck_person 0.106197 0.133709 0.7942 0.612481 0.1734
gamma_truck_guard -0.495867 0.089192 -5.5595 0.159593 -3.1071
gamma_age 0.009754 0.007375 1.3226 0.030696 0.3178
gamma_license -0.587134 0.177951 -3.2994 0.663174 -0.8853
gamma_k_tram -1.310026 0.150735 -8.6909 0.283321 -4.6238
gamma_k_social 1.778406 0.403119 4.4116 1.359554 1.3081
gamma_experi 0.885365 NaN NaN 0.765942 1.1559
gamma_video 1.724349 NaN NaN 0.659056 2.6164
gamma_none 1.935501 NaN NaN 0.829411 2.3336
gamma_ai_age -4.3736e-04 0.001051 -0.4162 0.002387 -0.1833
gamma_ai_license 0.088323 0.051582 1.7123 0.150062 0.5886
gamma_ai_k_tram 0.536744 0.054949 9.7680 0.192238 2.7921
gamma_ai_k_social 0.014143 0.047734 0.2963 0.079601 0.1777
gamma_ai_experi 1.568606 0.148065 10.5941 0.312211 5.0242
gamma_ai_video 0.749318 0.075226 9.9609 0.154114 4.8621
gamma_ai_none 0.674945 0.080731 8.3604 0.317445 2.1262
gamma_risk_age -0.006320 0.001390 -4.5465 0.004959 -1.2746
gamma_risk_license 0.080553 0.058921 1.3671 0.236654 0.3404
gamma_risk_experi 1.096366 0.079810 13.7372 0.209712 5.2280
gamma_risk_video 0.485445 0.078575 6.1781 0.275959 1.7591
gamma_risk_none 0.234845 0.091302 2.5722 0.316040 0.7431
tau_choice_1 -3.865454 0.160223 -24.1255 0.820664 -4.7102
tau_choice_2 -1.296618 0.133093 -9.7422 0.680984 -1.9040
tau_choice_3 1.257125 0.136315 9.2222 0.709085 1.7729
tau_choice_4 4.411921 0.169444 26.0376 0.842738 5.2352
zeta_ai_1 4.599298 0.273255 16.8315 0.392198 11.7270
zeta_ai_2 5.596781 0.372968 15.0061 0.654547 8.5506
zeta_ai_3 5.349914 0.349960 15.2872 0.540676 9.8949
zeta_ai_4 4.076730 0.234011 17.4211 0.377035 10.8126
tau_ai1_1 -5.145150 0.456335 -11.2749 0.923523 -5.5712
tau_ai1_2 -2.481426 0.306317 -8.1009 1.068569 -2.3222
tau_ai1_3 2.462841 0.291431 8.4509 1.057503 2.3289
tau_ai1_4 7.442935 0.454025 16.3932 1.107720 6.7192
tau_ai2_1 -6.245882 0.547194 -11.4144 0.762994 -8.1860
tau_ai2_2 -3.608538 0.385164 -9.3688 0.986890 -3.6565
tau_ai2_3 3.449865 0.412874 8.3557 1.635463 2.1094
tau_ai2_4 9.171504 0.672866 13.6305 2.197236 4.1741
tau_ai3_1 -5.777688 0.551108 -10.4838 1.330893 -4.3412
tau_ai3_2 -3.137507 0.375538 -8.3547 1.248736 -2.5125
tau_ai3_3 3.126005 0.355846 8.7847 1.270773 2.4599
tau_ai3_4 8.822468 0.582564 15.1442 1.448633 6.0902
tau_ai4_1 -4.714850 0.428471 -11.0039 1.089018 -4.3295
tau_ai4_2 -2.543842 0.295484 -8.6091 1.068058 -2.3817
tau_ai4_3 2.109538 0.259619 8.1255 0.985963 2.1396
tau_ai4_4 6.659229 0.391299 17.0183 1.101177 6.0474
zeta_risk_1 -0.447975 0.078522 -5.7051 0.116873 -3.8330
zeta_risk_2 -0.566177 0.087336 -6.4827 0.125780 -4.5013
zeta_risk_3 -0.580645 0.086111 -6.7430 0.120652 -4.8126
tau_risk1_1 -2.923248 0.141420 -20.6707 0.162163 -18.0266
tau_risk1_2 -1.930741 0.097631 -19.7759 0.129354 -14.9260
tau_risk1_3 -0.159030 0.067576 -2.3534 0.102127 -1.5572
tau_risk1_4 1.154220 0.077133 14.9640 0.102025 11.3132
tau_risk2_1 -3.659245 0.192346 -19.0243 0.196183 -18.6522
tau_risk2_2 -2.735413 0.131882 -20.7414 0.150007 -18.2353
tau_risk2_3 -1.386940 0.084472 -16.4189 0.106322 -13.0447
tau_risk2_4 0.668736 0.071209 9.3911 0.108679 6.1533
tau_risk3_1 -3.601368 0.186665 -19.2933 0.190539 -18.9010
tau_risk3_2 -2.463110 0.118944 -20.7082 0.142224 -17.3186
tau_risk3_3 -1.214904 0.081307 -14.9421 0.102600 -11.8411
tau_risk3_4 0.253863 0.068808 3.6894 0.101803
I ran three different datasets, but some show NaN in different values, in t-stat and SE.
I think this might be a problem of the code, and also I am not sure about the integrating latent variables and choice (ordered logit) part,
"Likelihood of choices" part, and generating multiple latent variables,
I will really appreciate your guidance,
Thank you in advance.
With best regards,
Hyewon
-
- Site Admin
- Posts: 1042
- Joined: 24 Apr 2020, 16:29
Re: How can I integrate ordered logit instead of MNL in ICLV?
Hyewon
this is a difficult model, and it makes it hard for us to diagnose where it goes wrong. My suggestion would be to start by estimating a model with the gammas fixed to zero and then gradually estimating those and seeing where it goes wrong
Stephane
this is a difficult model, and it makes it hard for us to diagnose where it goes wrong. My suggestion would be to start by estimating a model with the gammas fixed to zero and then gradually estimating those and seeing where it goes wrong
Stephane